Clusters Visualization
Clustering uses machine learning to identify the pattern of log records, and then to group the logs that have a similar pattern.
The Cluster view displays a summary banner at the top showing the following tabs:
-
Total Clusters: Total number of clusters for the selected log records.
Note
If you hover your cursor over this panel, then you can also see the number of log records (for example, 260 clusters from 1,413,036 log records).
-
Potential Issues: Number of clusters that have potential issues based on log records containing words such as error, fatal, exception, and so on.
-
Outliers: Number of clusters that have occurred only once during a given time period.
-
Trends: Number of unique trends during the time period. Many clusters may have the same trend. So, clicking this panel shows a cluster from each of the trends.
When you click any of the tabs, the histogram view of the cluster changes to display the records for the selected tab.
Each cluster pattern displays the following:
-
Trend: This column displays a sparkline representation of the trend (called trend shape) of the generation of log messages (of a cluster) based on the time range that you selected when you clustered the records. Each trend shape is identified by a Shape ID such as 1, 2, 3, and so on. This helps you to sort the clustered records on the basis of trend shapes.
Clicking the arrow to the left of a trend entry displays the time series visualization of the cluster results. This visualization shows how the log records in a cluster were spread out based on the time range that was selected in the query. The trend shape is a sparkline representation of the time series.
-
ID: This column lists the cluster ID. The ID is unique within the collection.
-
Count: This column lists the number of log records having the same message signature.
-
Sample Message: This column displays a sample log record from the message signature.
-
Log Source: This column lists the log sources that generated the messages of the cluster.
You can click Show Similar Trends to sort clusters in an ascending order of trend shapes. You can also select a cluster ID or multiple cluster IDs and click Show Records to display all the records for the selected IDs.
You can also hide a cluster message or multiple clusters from the cluster results if the output seems cluttered. Right-click the required cluster and select Hide Cluster.
In each record, the variable values are highlighted. You can view all the similar variables in each cluster by clicking a variable in the Sample Message section. Clicking the variables shows all the values (in the entire record set) for that particular variable.
In the Sample Message section, some cluster patterns display a <n> more samples... link. Clicking this link displays more clusters that look similar to the selected cluster pattern.
Clicking Back to Trends takes you back to the previous page with context (it scrolls back to where you selected the variable to drill down further). The browser back button also takes you back to the previous page; however, the context won’t be maintained, because the cluster command is executed again in that case.
Cluster the Log Data Using SQL Fields
The large volume of log records are reduced to 89 clusters, thus offering you fewer groups of log data to analyze.
You can drill down the clusters by selecting the variables. For example, from the above set of clusters, select the cluster that has the sample message SELECT version FROM V$INSTANCE
:
This displays the histogram visualization of the log records containing the specified sample message. You can now analyze the original log content. Click Back to Cluster to return to the cluster visualization.
The Trends panel shows the SQLs that have similar execution pattern.
The Outliers panel displays the SQLs that are rare and different.
Use Cluster Compare Utility
The cluster compare utility can be used to identify new issues by comparing the current set of clusters to a baseline and reducing the results by eliminating common or duplicate clusters. Some of the typical scenarios are:
- Which clusters are different in this week compared to last week?
- What's the difference between the cluster set of entity A and the set of entity B?
- Things were working well in the month X. What changed in this month?
Given two sets of log data, the cluster compare utility removes the data pertaining to the common clusters, and displays histogram data and the records table that are unique to each set. For example, when you compare the log data from week x and week y, the clusters that are common to both the weeks are removed for simplification, and the data unique to each week is displayed. This enables you to identify patterns that are unique for the specific week, and analyze the behavior.
For the syntax and other details of the clustercompare
command, see
clustercompare.
- In clusters
visualization, select your current time range. By default, the query is
*
. You can refine the query to filter the log data. - In the Visualize panel, click Cluster
Compare.
The cluster compare dialog box opens.
- You can notice that the current query and the current time range are displayed for reference.
- Baseline Query: By default, this is the same as your current query. Click the
and modify the baseline query, if required.
- Baseline Time Range: By default, the cluster compare utility uses the Use Time Shift option to determine the baseline time range. Hence, the baseline time range is of the same duration as the current time range and is shifted to the period before the current time range. You can modify this by clicking the
icon and selecting Use Custom Time or Use Current Time. If you select Use Custom Time, then specify the custom time range using the menu.
- Click Compare.
You can now view the cluster comparison between the two log sets.
Click the button corresponding to each set to view the details like clusters, potential issues, outliers, trends, and records table that are unique to the set. The page also displays the number of clusters that are common between the two log sets.
In the above example, there are 11 clusters found only in the current range, 4 clusters found only in the baseline range, and 30 clusters common in both the ranges. The histogram for the current time range displays the visualization using only the log data that is unique to the current time range.
- Baseline Query: By default, this is the same as your current query. Click the
Clusters found only in the current range are returned first, followed by clusters found only in the baseline range. The combined results are limited to 500 clusters. To reduce the cluster compare results, reduce the current time range or append a command to limit the number of results. For example, append | head 250
will limit both current and baseline clusters to 250 each. Use multi-select (click and drag hold) on the cluster histogram to reduce the current time range when using the custom time option. The time range shift value may be converted to minutes or seconds to ensure no time gaps or overlaps occur between the current and baseline time ranges.
Use Dictionary Lookup in Cluster
Use dictionary lookup after the cluster
command to annotate
clusters.
Consider the cluster
results for Linux Syslog Logs. To
define a dictionary to add labels based on the Cluster Sample field:
-
Create a CSV file with the following contents:
Operator,Condition,Issue,Area CONTAINS IGNORE CASE,invalid compare operation,Compare Error,Unknown CONTAINS IGNORE CASE REGEX,DNS-SD.*?Daemon not running,DNS Daemon Down,DNS CONTAINS ONE OF REGEXES,"[[Cc]onnection refused,[Cc]onnection .*? closed]",Connection Error,Network CONTAINS IGNORE CASE,syntax error,Syntax Error,Validation CONTAINS IGNORE CASE REGEX,Sense.*?(?:Error|fail),Disk Sensing Error,Disk CONTAINS IGNORE CASE REGEX,device.*?check failed,Device Error,Disk
Import this as a Dictionary type lookup using the name Linux Error Categories. This lookup contains two fields, Issue and Area that can be returned on a matching condition. See Create a Dictionary Lookup.
-
Use the dictionary in cluster to return a field:
Run the
cluster
command for Linux Syslog Logs. Add alookup
command aftercluster
, as shown below:'Log Source' = 'Linux Syslog Logs' | cluster | lookup table = 'Linux Error Categories' select Issue using 'Cluster Sample'
The value of Cluster Sample for each row is evaluated against the rules defined in the Linux Error Categories dictionary. The Issue field is returned from each matching row.
-
Return more than one field by selecting each field in the
lookup
command:'Log Source' = 'Linux Syslog Logs' | cluster | lookup table = 'Linux Error Categories' select Issue as Category, Area using 'Cluster Sample'
The above query selects the Issue field, and also renames it to Category. Area field is also selected, but not renamed.
-
Filter the cluster results using the dictionary fields:
Use the
where
command on the specific fields to filter the clusters. Consider the following query:'Log Source' = 'Linux Syslog Logs' | cluster | lookup table = 'Linux Error Categories' select Issue as Category, Area using 'Cluster Sample' | where Area in (Unknown, Disk)
This displays only those records that matches the specified values for Area field.
Semantic Clustering
Cluster Visualization allows you to cluster text messages in log records. Cluster works by grouping messages that have similar number of words in a sentence, and identifying the words that change within those sentences. Cluster does not consider the literal meaning of the words during the grouping.
The nlp
command supports semantic clustering. Semantic
Clustering is done by extracting the relevant keywords from a message and clustering
based on these keywords. Two sets of messages that have similar words are grouped
together. Each such group is given a deterministic Cluster ID.
The following example shows the usage of NLP clustering and keywords on Linux Syslog Logs:
'Log Source' = 'Linux Syslogs Logs'
| link Time, Entity, cluster()
| nlp cluster('Cluster Sample') as 'Cluster ID',
keywords('Cluster Sample') as Keywords
| classify 'Start Time', Keywords, Count, Entity as 'Cluster Keywords'
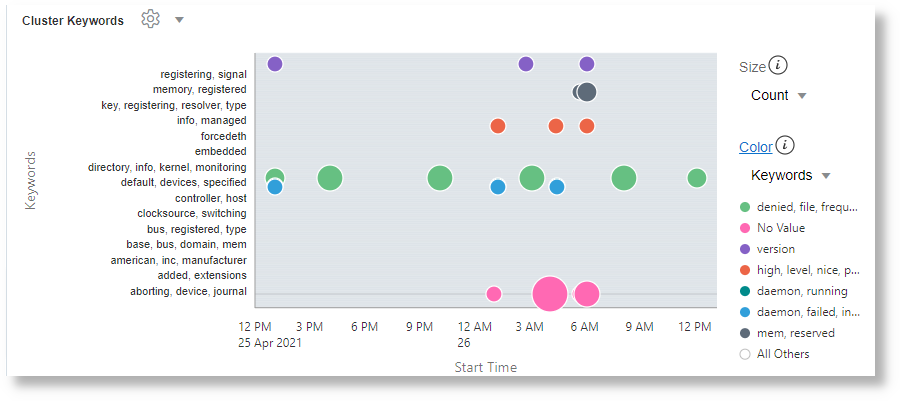
For more example use cases of semantic clustering, see Examples of Semantic Clustering.
nlp
Command
The nlp
command can be used only after the
link
command and supports two functions.
cluster()
can be used to cluster the specified field, and
keywords()
can be used to extract keywords from the specified
field.
nlp
command can be used only after the
link
command. See nlp.
-
nlp cluster()
:cluster()
takes the name of a field generated in Link, and returns a Cluster ID for each clustered value. The returned Cluster ID is a number, represented as a string. The Cluster ID can be used in queries to filter the clusters.For example:
nlp cluster('Description') as 'Description ID'
- This would extract relevant keywords from theDescription
field. TheDescription ID
field would contain a unique ID for each generated cluster. -
nlp keywords()
:Extracts keywords from the specified field values. The keywords are extracted based on a dictionary. The dictionary name can be supplied using the
table
option. If no dictionary is provided, the out-of-the-box default dictionary NLP General Dictionary is used.For example:
nlp keywords('Description') as Summary
- This would extract relevant keywords from theDescription
field. The keywords are accessible using theSummary
field.nlp table='My Issues' cluster('Description') as 'Description ID'
- Instead of the default dictionary, use the custom dictionary My Issues.
NLP Dictionary
Semantic Clustering works by splitting a message into words, extracting the relevant words and then grouping the messages that have similar words. The quality of clustering thus depends on the relevance of the keywords extracted.
- A dictionary is used to decide what words in a message should be extracted.
- The order of items in the dictionary is important. An item in the first row has higher ranking than the item in the second row.
- A dictionary is created as a .csv file, and imported using the Lookup user interface with Dictionary Type option.
- It is not necessary to create a dictionary, unless you want to
change the ranking of words. The default out-of-the-box
NLP General Dictionary
is used if no dictionary is specified. It contains pre-trained English words.
See Create a Dictionary Lookup.
Following is an example dictionary iSCSI Errors:
Operator | Condition | Value |
---|---|---|
|
error |
noun |
|
reported |
verb |
|
iSCSI |
noun |
|
connection |
noun |
|
closed |
verb |
The first field is reserved for future use. Second field is a word. The
third word specifies the type for that word. The type can be any string and can be
referred to from the query using the category
parameter.
In the above example, the word error has higher ranking than the words reported or iSCSI. Similarly, connection has higher ranking than closed.
Using a Dictionary
Suppose that the following text is seen in the Message
field:
Kernel reported iSCSI connection 1:0 error (1020 - ISCSI_ERR_TCP_CONN_CLOSE: TCP connection closed) state (2) Please verify the storage and network connection for additional faults
The above message is parsed and split into words. Non-alphabets are removed. Following are some of the unique words generated from the split:
Kernel reported iSCSI connection error ERR TCP CONN CLOSE closed state ... ...
There are a total of 24 words in the message. By default, semantic clustering would attempt to extract 20 words and use these words to perform clustering. In a case like the above, the system needs to know which words are important. This is achieved by using the dictionary.
The dictionary is an ordered list. If iSCSI Errors is used, then NLP would not extract ERR, TCP, or CONN because these words are not included in the dictionary. Similarly, the words error, reported, iSCSI, connection, and closed are given higher priority due to their ranking in the dictionary.